Artificial Intelligence Medicine
Overview
A large amount of big data has been produced in the fields of medicine and biology due to advances in measurement technology. Our laboratory is conducting research with the goal of establishing a mathematical/infomatics foundation for understanding life phenomena and applying them to predictive and personalized medicine.
The three main research themes are 1. Identification of regulatory factors based on biological network, 2. Stratification and translational research using machine learning, 3. State transition modeling and future prediction of chronic diseases.
Professor:
Eiryo Kawakami
TEL: +81-43-226-2826
FAX: +81-43-226-2826
e-mail: eiryo.kawakami●chiba-u.jp
URL: http://www.m.chiba-u.ac.jp/dept/chiba_ai/
※ Please change "●" mark to at-mark if you send emails.
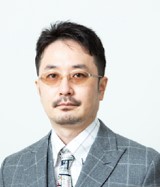
Research & Education
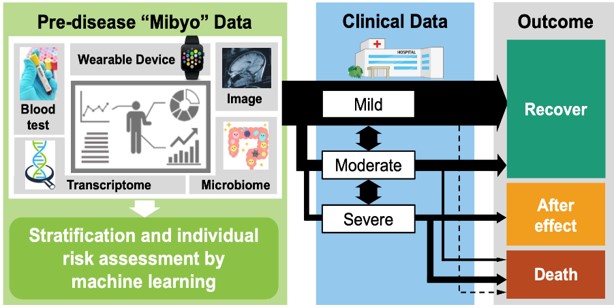
Artificial Intelligence (AI) is capable of making highly accurate predictions of diseases that outperform experts based on high-dimensional data. On the other hand, there are cases where the classifications of diseases to be predicted are unclear and prediction is inherently difficult. In such cases, unsupervised learning can be used to stratify life phenomena and diseases to find classifications that even experts have not noticed before1,2. In addition, chronic diseases take a long-term course, and once they develop, it is difficult to recover to a healthy state. Therefore, it is more important to prevent serious changes before they occur. We are developing technologies for convenient monitoring of disease status in daily clinical practice3, as well as for stratification and individual risk assessment based on pre-disease, or “Mibyo”, data. At the School of Medicine we are responsible for teaching the fundamentals of machine learning and data science; through hands-on programming in Python and R, we help students learn data handling and application of machine learning.
Recent Publications
- Eiryo Kawakami, Junya Tabata, Nozomu Yanaihara, Tetsuo Ishikawa, Keita Koseki, Yasushi Iida, Misato Saito, Hiromi Komazaki, Jason S Shapiro, Chihiro Goto, Yuka Akiyama, Ryosuke Saito, Motoaki Saito, Hirokuni Takano, Kyosuke Yamada, Aikou Okamoto. Application of Artificial Intelligence for Preoperative Diagnostic and Prognostic Prediction in Epithelial Ovarian Cancer Based on Blood Biomarkers. Clin cancer Res. 4 25(10) 3006-3015, 2019
- Kiwako Yamamoto-Hanada, Eiryo Kawakami, Mayako Saito-Abe, Miori Sato, Hiroshi Mitsubuchi, Masako Oda, Takahiko Katoh, Masafumi Sanefuji, Shouichi Ohga, Mari Kuwajima, Nathan Mise, Akihiko Ikegami, Fujio Kayama, Ayako Senju, Masayuki Shimono, Koichi Kusuhara, Shin Yamazaki, Shoji F Nakayama, Kenji Matsumoto, Hirohisa Saito, Yukihiro Ohya. Exploratory analysis of plasma cytokine/chemokine levels in 6-year-old children from a birth cohort study. Cytokine 130:155051, 2020
- Keita Koseki, Hiroshi Kawasaki, Toru Atsugi, Miki Nakanishi, Makoto Mizuno, Eiji Naru, Tamotsu Ebihara, Masayuki Amagai, Eiryo Kawakami. Assessment of skin barrier function using skin images with topological data analysis. NPJ systems biology and applications 6(1) 40 - 40, 2020